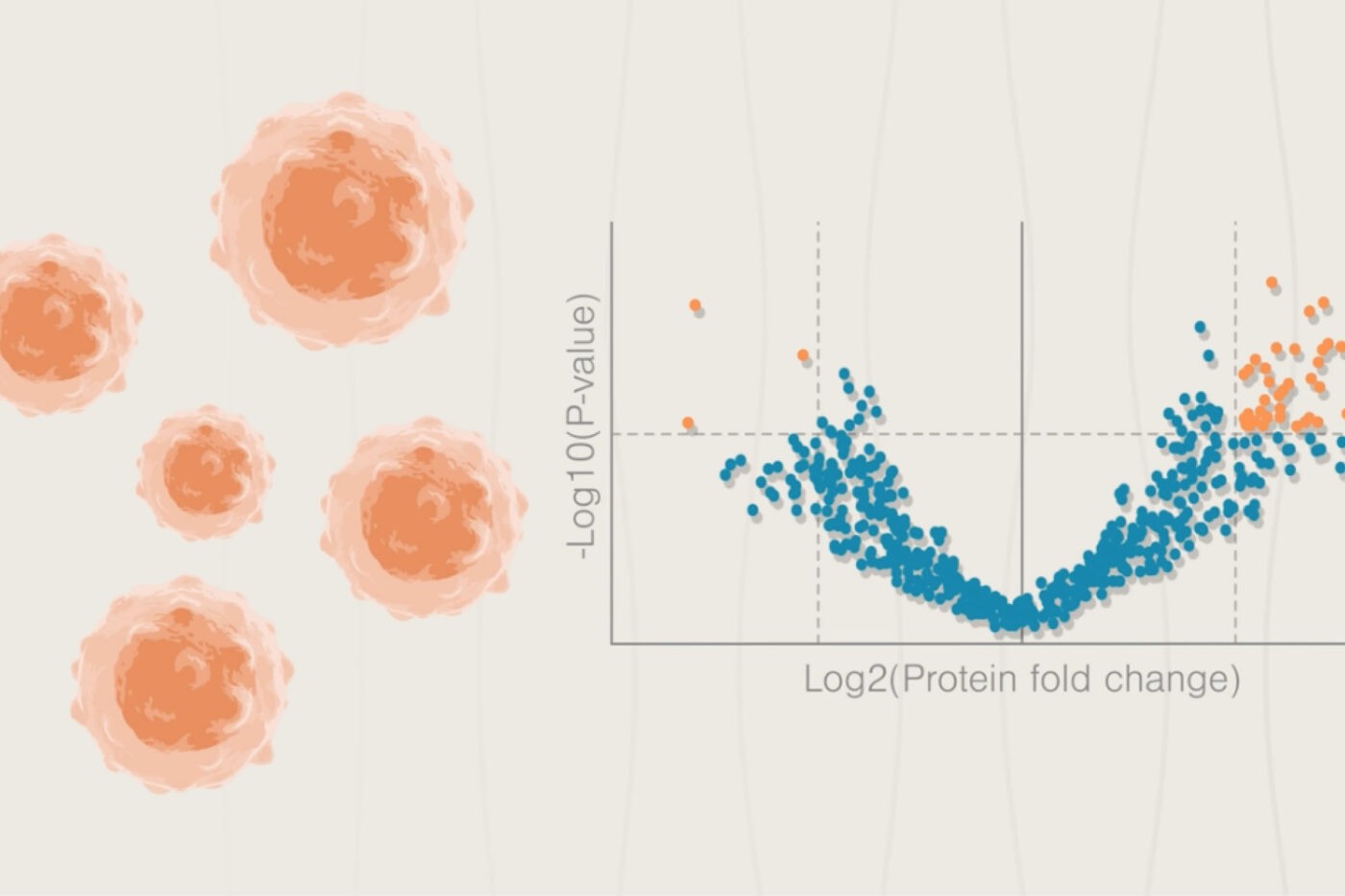
Using proteomics to expand the scope of cancer research beyond driver mutations
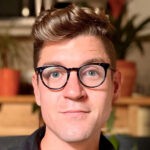
Tyler Ford
February 22, 2024
While the genome encodes all the proteins a cell can make, it does not reveal protein abundance, show you how proteins will be post-transcriptionally modified, or show you how those proteins will interact to achieve complex functions. Proteome profiling, on the other hand, shows researchers what functions and malfunctions are active in cells now. Thus, proteomics is much better suited to revealing what’s going wrong in a cell than genomics.
There is perhaps no condition where this is truer than cancer. Cancer researchers have focused much of their effort on finding “driver mutations” using genomics. These alterations in DNA enable cancer cells to develop. Driver mutations can cause the initial events that set cells on a cancerous path or give cancer cells additional selective advantages at later stages of malignancy.
Finding driver mutations helps researchers understand the natural history of cancer cells and can point to therapeutic targets, but multiomics studies consistently show driver mutations are only the beginning of the story. Focusing too much on driver mutations can lead to red herrings in cancer research and drug discovery.
There are many reasons for this, but broadly speaking, biology functions at the level of proteins, not genes. Furthermore, protein abundance and activity are modified in many ways that are not enacted at the level of genes. For example:
- Transcript levels can increase or decrease thereby altering protein abundance
- Translation rates can increase or decrease thereby altering protein abundance
- Protein degradation rates can increase or decrease thereby altering protein abundance
- Alternative transcripts and spliceoforms can be generated thereby altering protein function and abundance
- Proteins can be modified through the activity of inteins, kinases, gylcosylases, and much more
As a result of these and other activities, the functional proteoforms present in a cell can be far removed from what you might expect by looking at DNA. One straightforward example of this is in the effects of gene copy number variation. Things like chromosomal translocations and other errors can duplicate genes or delete them. These events can drive the initial processes that give rise to cancer, but protein abundance often fails to correlate with gene dosage. I.e. duplicating a gene does not mean protein abundance doubles.
One might posit (and many have) that measuring mRNA abundance is a better proxy for, at the very least, protein levels. Yet, study after study shows that protein levels often correlate poorly with transcript levels. Compounding the problem, protein activity doesn’t always correlate with protein abundance either.
Thus, it’s clear that researchers must look to proteins to understand cellular function. Given that cancer cells undergo rapid proliferation and continuous change, this is very apparent in cancer. Mutations in DNA set cells on a malignant path, but downstream changes to protein levels can alter metastasis, drug resistance, recurrence, and more. Below we dive into a few specific examples.
Watch this animation to discover some of the many things proteomics can reveal about cancer:
Proteomic changes driving cancer recurrence in glioblastoma
Kim et al. 2024 recently showed that primary glioblastomas often have mutations in genes controlling proliferation, but these are not as prevalent in recurrent, treatment-resistant glioblastoma. Instead, a different set of abundant and active proteins give recurrent glioblastomas characteristics of neuronal cells and make them resistant to frontline therapies. These proteins often have increased protein or phosphoprotein abundance without highly correlated changes in transcript abundance.
Furthermore, while initial driver-mutation encoded proteins may be elevated in primary tumors, they can decrease in abundance or have lower activity in recurrent tumors. Sometimes initial driver mutations may even be lost in the recurrent tumor. Rather than focusing on these “driver genes” in the recurrent tumors, Kim et al. identified proteins to target therapeutically in recurrent glioblastomas using proteomics. In particular, their proteomic analysis showed that BRAF had increased activity in recurrent tumors. Later, when the researchers treated mouse models of recurrent glioblastoma with a BRAF inhibitor, they survived for longer and had smaller tumors than controls.
Mutations alone do not demonstrate functional biology
Mutations may be necessary to set cells on the path to malignancy, but once on that path, a wide variety of processes can alter protein levels in ways that lead to resistance and recurrence. Some resistance mechanisms will be genetically based, but even those that are will have effects that are difficult to sus out amongst a background of high mutation rates. Proteomics thereby has the potential to identify the salient downstream effects that lead to resistance and recurrence at the functional level of proteins.
Tumor microenvironment proteomes change as a result of HNSCC metastasis
Studying tumor microenvironmental proteomes in head and neck squamous cell carcinoma cells, Busso-Lopes et al. show that non-malignant cells have altered protein abundance between patients with and without metastases. These differences could not have been predicted from genomics and driver mutation data alone. Mutations in cancer cells simply do not tell you how non-malignant cells respond to those changes. Furthermore, while it is unlikely that the genomes of the non-malignant cells were altered (this wasn’t assessed in this study), there are many ways that cancer cells can alter the proteomes of non-malignant cells. Importantly, both the proteomes of malignant and non-malignant cells are subject to therapeutic intervention.
Assessing changes to the tumor microenvironment requires proteomics
Overall, any time researchers study differences between non-cancerous cells in the various tumor microenvironments of one patient or between patients, it will be more informative to conduct proteomic analyses than genomic analyses. There may be rare mutations in non-cancerous cells that make a patient particularly prone to something like metastasis, but a proteomic analysis will pick up both the effects of such rare mutations and any significant differences that are not genetically based.
Proteomic differences in responders and non-responders to immunotherapy
Harel et al. investigated proteomic differences between melanoma patients who did and did not respond to two types of immunotherapy: tumor infiltrating lymphocyte-based or checkpoint inhibitor-based immunotherapy. In both cases, tumors from responders had marked increases in metabolic proteins associated with mitochondrial metabolism and increases in proteins involved with antigen presentation. The researchers go on to show that activating mitochondrial metabolism using a small molecule increased the expression of the antigen presentation machinery in melanoma cell lines. Furthermore, using CRISPR to knock out genes driving mitochondrial metabolism in melanoma cell lines enhanced their growth and decreased the abundance of proteins involved in antigen presentation.
The authors use their proteomic data to identify signatures of responders and non-responders and note that previous efforts to identify such signatures using histological or genomic approaches were not effective. Although historical data did show similar changes in the transcripts encoding the signature proteins, these were not as prominent as the changes in protein abundances.
Proteomics enables the development of targeted therapies for cancer
In the age of precision medicine, it is critical to identify patients who will likely respond to targeted therapies. This study makes it clear that proteomics, more than genomics or transcriptomics, can provide clear signatures of responsiveness that can help physicians direct patients to the most effective therapies.
Moving beyond driver mutations in the proteomics era
Identifying driver mutations can help researchers understand the biology underlying cancer development, but focusing too much on driver mutations may cause researchers to overlook downstream changes that are proximal enablers of cancer cell growth, metastasis, drug resistance, immune evasion, and more. Only by placing driver mutations in a multiomic context that heavily relies on proteomic data to understand what’s actually happening in cancer cells in real time, can researchers and physicians identify the best ways to treat an individual’s cancer. As we develop next generation proteomics technologies that make proteomics accessible, we hope to enable scientists to uncover the intricacies of cancer and enable physicians to leverage those intricacies for novel and more effective treatments.
MORE ARTICLES
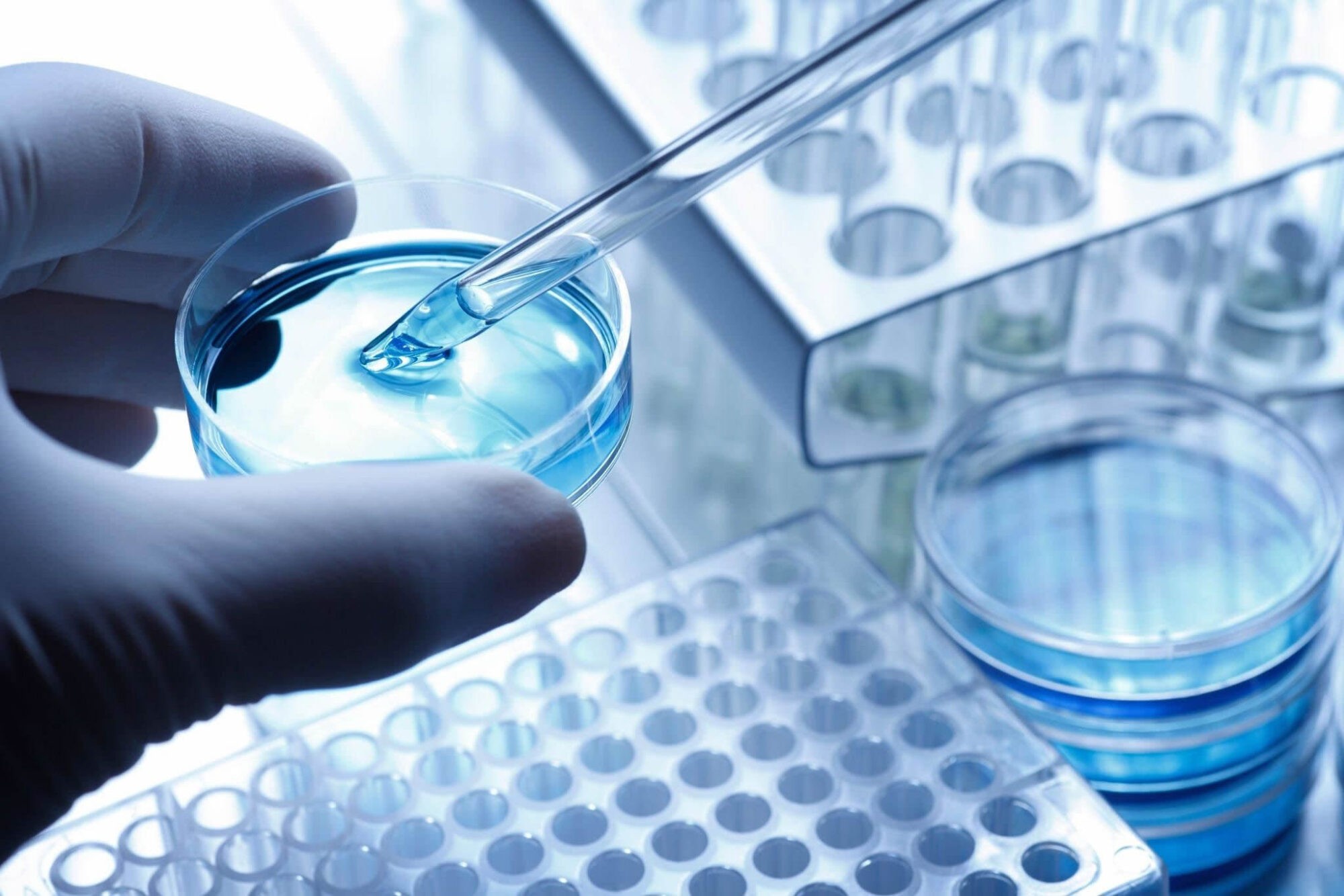