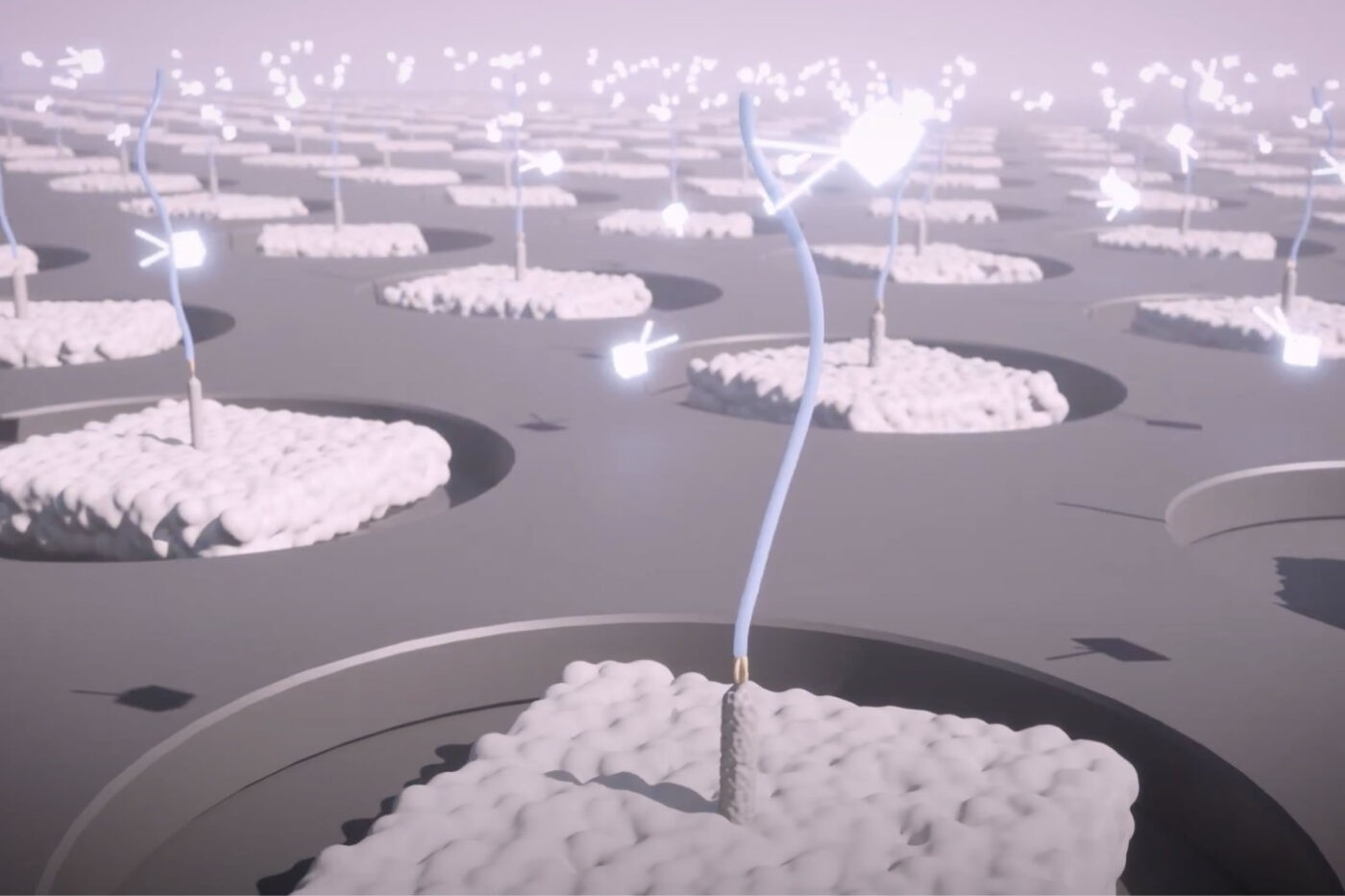
Protein “Guess Who I Am” – How the Nautilus Platform is designed to quantify billions of proteins at the single-molecule level
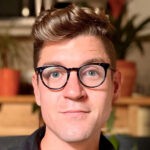
Tyler Ford
January 9, 2024
When aiming to achieve any goal, there are always more and less efficient options. For instance, you could mow your lawn by snipping each blade of grass with scissors, or you could do the job quickly and effortlessly with a ride-on mower. The same is true in proteomics. You could painstakingly identify every protein in the proteome one-by-one, or you could develop a technique that interrogates all proteins in the proteome simultaneously.
We have taken the later tactic and designed our platform to efficiently quantify substantively the entire proteome in broadscale discovery proteomics experiments. The platform makes use of a strategy similar to one you might use to win the popular children’s game “Guess Who I Am.”
Read on to learn how our platform leverages protein “Guess Who I Am” for efficient proteome-wide protein quantification and check out our recent animation below for a visual deep dive into the quantification process.
https://youtu.be/aZsQSdFpb4A?si=kw3UNiYs7FNv8OYT
Quantifying billions of proteins at the single-molecule level simultaneously
Our single-molecule proteomics methodology is designed to enable the Nautilus Proteome Analysis Platform to quantify proteins across the wide dynamic range of the proteome. Using hyper-dense protein arrays, we simultaneously isolate billions of individual protein molecules and repeatedly interrogate them using multi-affinity probes. The Nautilus Platform uses binding information to identify each distinct molecule and we add up all proteins with a given identity to quantify them. In other words, our platform conducts single-molecule analysis at the scale of the proteome.
There are two conceptual ways to achieve full proteome coverage with this approach. Each corresponds to a strategy in “Guess Who I Am.” In this popular children’s game, it is your goal to identify one character from a large group of characters by asking as few questions about them as possible and eliminating possibilities based on answers about the target character. Similarly, on our platform, the goal is to identify each protein on each landing pad by probing its characteristics and eliminating possibilities based on these characteristics.
- Strategy 1 (The inefficient way) – Create affinity probes specific to each genetically-encoded protein (~20,000 in humans). This is like asking one question for each character in “Guess Who I Am.” In the game, this strategy would only enable you to eliminate one character per round. In the proteomics experiment, it forces you to identify each protein species on the array one at a time.
- Strategy 2 (The efficient way) – Create non-specific multi-affinity probes that bind to features of many proteins, build information about many proteins at once, and use far fewer than 20,000 reagents to cover 20,000 genetically-encoded proteins. This is like asking broad questions that relate to many characters on the “Guess Who I Am” board. In the game, this strategy eliminates many possible characters at once. In the proteomics experiment, it eliminates many possible identities and quickly identifies all protein species at the same time.
Let’s dive into each strategy to get a better feel for just how different they are.
The inefficient way to quantify proteins – Specific probes for every protein
Assuming you could make probes specific to every genetically-encoded protein, there are very practical reasons this technique is inefficient – time and expense.
This approach would require 20,000 cycles of probe binding to identify every human protein species on the array. This would likely translate into weeks for a single run. Not only would this take valuable time, but the expense of using that many reagents would be astronomical.
For this strategy, you would also need to generate probes that are specific for their targets and even a small amount of off-target binding could lead to substantial inaccuracies in the data. Unfortunately, creating such probes is no easy task, and traditional affinity probes are notoriously promiscuous (think about those mystery bands on western blots generated using supposedly specific antibodies).
Using a similar strategy in “Guess Who I Am” would probably cause you to lose. Using it in a research setting would waste time and money, increase your time to publication, and make scientists unhappy.
The efficient way to quantify proteins – nonspecific multi-affinity probes
To get around these issues, we’ve developed a methodology that we call Protein Identification by Short epitope Mapping or PrISM. Instead of using 20,000 highly specific probes, this methodology leverages hundreds of non-specific multi-affinity probes that bind to short amino acid sequences shared across many proteins. When we flow these probes across the billions of single protein molecules on our arrays, they are designed to provide information about every protein at once. Through iterative cycles, enough information is acquired to distinguish all protein species.
Like broad questions in “Guess Who I Am,” our multi-affinity probes rapidly eliminate many possible identities for each single protein molecule through a relatively small number of cycles of probe binding. In fact, our computational modeling shows that it should only take 300 cycles of multi-affinity probe binding to confidently identify substantively all proteins in the proteome. That’s far fewer than the equivalent experiment with specific probes.
The similar strategy in “Guess Who I Am” would likely lead to victory. In research it may lead to more rapid insight generation, faster publication, and happier scientists.
Stochasticity in multi-affinity probe binding
Of course, biology is more complicated than “Guess Who I Am.” We can never be sure that our multi-affinity probes will bind to their target epitopes on every protein molecule in every cycle. Instead, each probe is characterized with a predicted binding probability that is refined through machine learning.
When you get into the nuts and bolts of multi-affinity probe binding, its probabilistic nature means you will not get the same pattern for every instance of a protein species across the array or across multiple runs. There is no “signature” pattern for a protein species.
Instead, our decoding algorithm presents a likelihood of a given protein identity based on known affinity probe binding probabilities, known protein sequences, and the patterns observed at each landing pad. In fact, the algorithm does not rely on any single multi-affinity probe for its identifications. Instead, it incorporates information built over all 300 cycles and no single cycle or probe is essential for proper identification. Thus, even if some probes fail to bind or there are some errors in some cycles, ultimate identifications are unaffected. The result is a platform that generates robust and reproducible data at scale.
In this sense, the strategy used to identify proteins on the platform is more flexible than the “broad questions” strategy in “Guess Who I Am.” Our questions (multi-affinity probes) don’t need to be perfect. They need to be just good enough to build a bit more information about the proteins across the array.
Learn more about protein quantification on the NautilusTM Proteome Analysis Platform
Hyper-dense arrays, multi-affinity probes, our PrISM methodology, and advanced machine learning culminate in a platform designed to quantify proteins robustly and reproducibly across substantively the entire proteome. If you’d like to learn more about our decode process, check out our PrISM preprint and tech note.
MORE ARTICLES
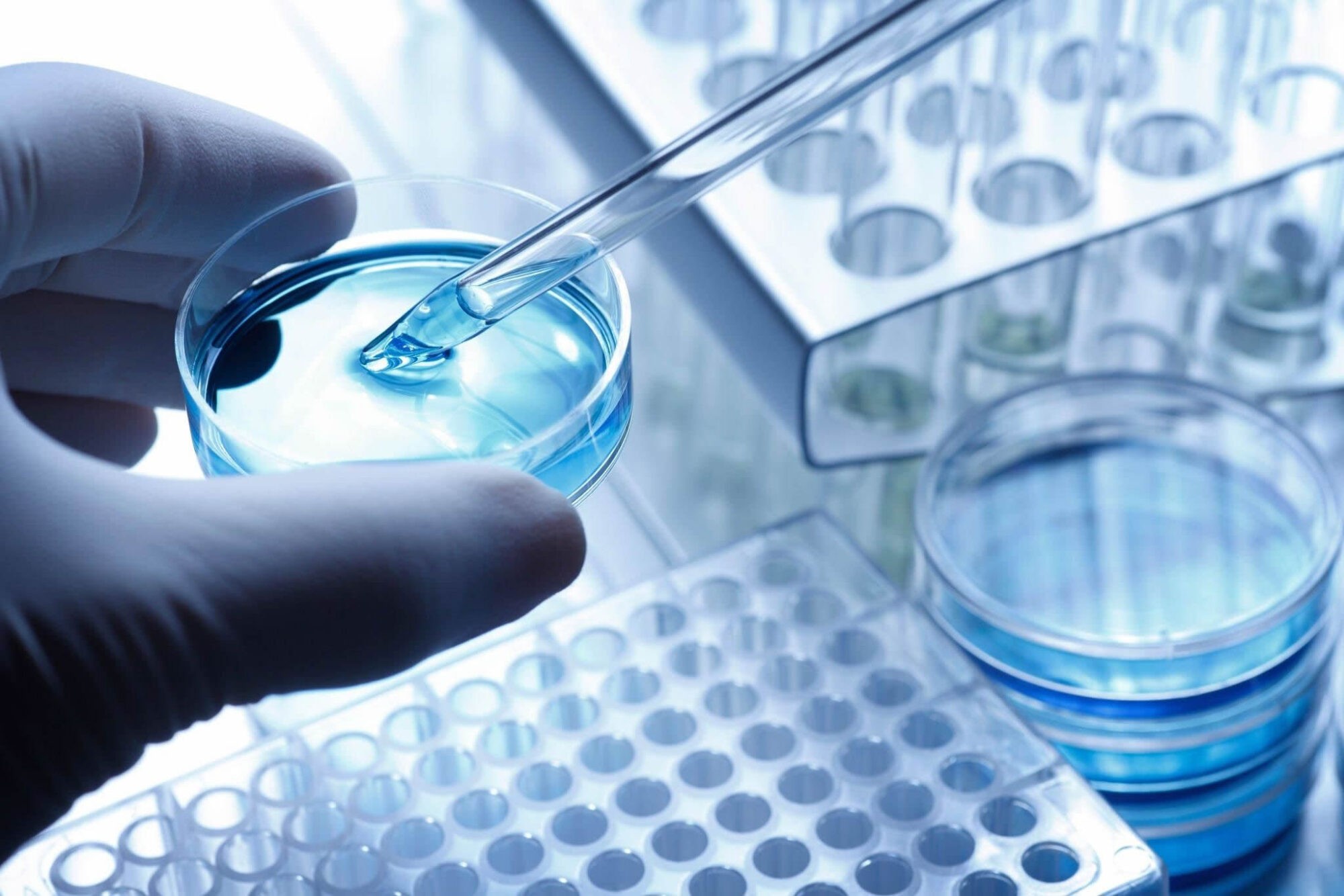