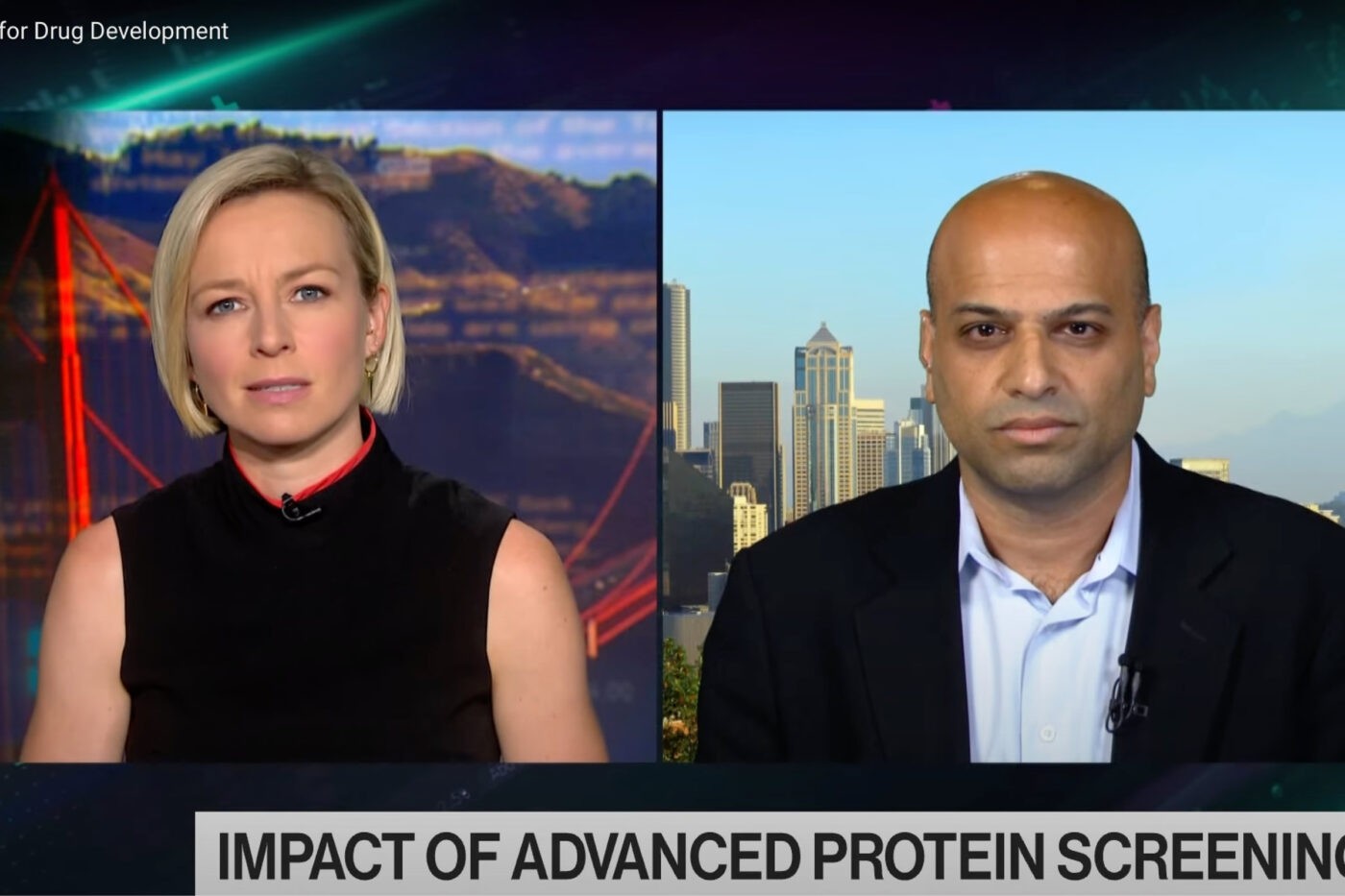
Congratulations to Nautilus Founder and Chief Scientist, Parag Mallick, winner of the 2024 US HUPO Gilbert S. Omenn Computational Proteomics Award
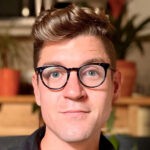
Tyler Ford
December 6, 2023
Nautilus Chief Scientist, Parag Mallick, is a great example of a cross functional leader. He has experience in molecular biology, biochemistry, mass spectrometry, graphic design, and, of all things, magic. Rounding out his diverse interests, Parag is also an accomplished computational biologist, and the Human Proteome Organization (HUPO) recently recognized this fact by choosing Parag as the recipient of the 2024 US HUPO Gilbert S. Omenn Computational Proteomics Award!
Read the interview below to learn about Parag’s foundational impacts in computational proteomics and discover how his expertise influences technology development at Nautilus.
Find Parag’s full 2024 US HUPO Gilbert S. Omenn Computational Proteomics Award lecture here.
Why did you start working in computational biology/proteomics?
My interest in biology and computer science started very early. The combined influences of mentors in high school and at Space Camp provided the foundations for my interest in computational biology and later focused me on work at the intersection of proteins and computing more specifically. My high school biology teacher, Diane Sweeney, was an incredibly passionate scientist who worked in biotech as a molecular biologist prior to teaching while my instructor in mathematics and computing, Mark Lawton, previously worked on problems in medical imaging. Their lessons inspired interests in biology and computing. Later, a counselor at Space Camp sparked my fascination with proteins by describing the ways these fundamental molecular machines contribute to calcium regulation and bone demineralization, a common problem for astronauts. During conversations in the science bay of a model spaceship, he viscerally tied the macro-scale problem of weakening bones to the molecular workings of proteins and clued me in to the ways computers could help us better understand protein structure and function to solve biological problems – inspiring me to learn more about computational biology.
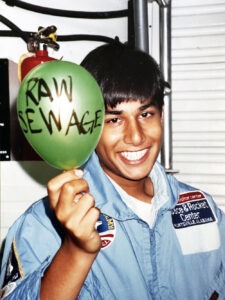
Parag Mallick at Space Camp
Animated by the unique perspectives of these mentors, I chose to study biochemistry, mathematics and computer science at Washington University in St. Louis. At Wash U and in the Eisenberg lab at UCLA, I had the privilege of conducting undergraduate and graduate research on protein fold and function at proteome scale. These studies led me to appreciate biology as a complex system where cellular and organismal behavior is driven by complex networks of molecules working synergistically. Throughout my training, I was fortunate to be in environments where computation worked hand-in-hand with bench-top experimentation to accomplish things neither could on their own. Since then, the combined forces of computation and bench top experimentation have fueled my career.
Towards the end of my graduate studies at UCLA, the universe seemed to conspire to guide me to mass spectrometry-based proteomics. In one of my graduate projects, we used 2D gels and mass spectrometry to investigate disulfide bonds at proteome-scale. At the same time, my sister, a cancer surgeon, sent me what she considered a game-changing paper describing how mass spectrometry might be used to diagnose cancer. Later, I serendipitously got the opportunity to meet with some of the foremost leaders in the field – Joe Loo joined the UCLA faculty, and both Ruedi Aebersold and Matthias Mann came to UCLA for a symposium. My graduate advisor had pointed to both Ruedi and Matthias as potential postdoc mentors and I was incredibly excited when Ruedi later welcomed me into his lab as a postdoctoral fellow.
My time in Ruedi’s lab made it clear that mass spectrometry-based quantitative measurements of protein abundance could generate tremendous amounts of data. With these quantitative measures of the proteome, we could better understand everything from the fundamentals of biochemistry to the depths of cellular regulation and dysregulation that dictate health and disease. As I dove deeper into the technical aspects of proteomics, I always kept the big picture questions from my Space Camp days in the back of my mind – How could proteomics help us understand what happens to astronauts in space? How could protein-based “big data” reveal the innerworkings of biology and better relate function at the molecular level to phenotype? Crossing these biological scales was and is incredibly exciting.
Find Parag on our Translating Proteomics podcast
What have been some of your biggest accomplishments in the computational proteomics space?
I’m very excited about the applied work my lab does using multiomics strategies to better understand cancer as a complex system and uncover novel biomarkers that might one day help us diagnose cancers earlier and lead to better patient outcomes.
However, anyone who works in proteomics knows that mass spectrometry workflows and data can be…complex. Additionally, it’s not yet clear how best to integrate proteomics data with other modalities. I’ve always felt that there are lots of ways to have impact and to make the world a better place. Some of that can come through detailed mechanistic studies that help us understand the natural world, where diseases come from, and what to do about them. However, tools that make science easier, faster, more accessible, more reproducible, and more efficient can also have tremendous impact. Consequently, a ton of my work has focused on making proteomics more accessible, on developing approaches for linking data across omics levels and, more generally, making it easier for the broader scientific community to get from data to insight.
Perhaps my most impactful work in this area has been leading the development of ProteoWizard with the help of my many amazing students and collaborators – particularly Darren Kessner, with whom I created the very first implementation of ProteoWizard, Matt Chambers from the Tabb Lab, and Brendan Maclean from the MacCoss Lab (and the dozens and dozens of additional developers who have contributed to the project). ProteoWizard is a set of open source software libraries that enable researchers to read and convert raw data from popular mass spectrometry instruments into a standard format that can be compared and analyzed across labs. Additional software libraries reduce the barrier to developing novel software by providing the foundational methods required by most analysis pipelines.
What spurred you to create ProteoWizard?
ProteoWizard first came into existence when my student, Darren Kessner, was developing tools to help our lab interpret some of our own mass spectrometry data. As we were working together, it was clear there were substantial barriers to entry into the field of proteomics data analysis. Our thought was, if we could lower or remove those barriers, it might generally accelerate the development of novel proteomics analysis approaches – not just for our lab – but for the whole field.
To give you a sense of the world back then – before one could even consider creating advanced analytics approaches, a lot of “boring” code had to be built (such as parsers, peptide mass calculators, etc.). Additionally, mass spectrometry platforms from different vendors often output data files that could only be analyzed using the proprietary software that came with their instruments. This was a tremendous barrier to open science, data sharing, and reproducibility. Furthermore, most bioinformatics researchers didn’t own instruments – and so they couldn’t even begin to develop novel analytic methods. Imagine a world where pictures you took on your Go Pro could only be viewed or manipulated on a Go Pro and where there were no accessible software tools for editing those images. That’s the world we were in.
Darren was an exceptional software developer who had previously worked at places like Symantec and Sony before coming to my lab. After discussing our options for how to tackle this problem, not just for ourselves, but for the whole field, we decided to create reliable proteomics software built on a foundation of modern software development principles. Importantly, this software would be portable between labs and relatively “easy” to use. We soon found exceptional partners to help us create these tools and built a community of dozens of developers who contributed code to the project.
This effort required buy-in from researchers in the proteomics community as well as mass spectrometry manufacturers who were tied to their proprietary analysis tools. There was a lot of inertia against us, but we pushed forward and successfully created a flexible software suite that could be licensed to academic and industry researchers and intake raw data from popular mass spectrometry vendors.
Since then, ProteoWizard has become a mainstay of mass spectrometry analysis. Through collaborative efforts with the MacCoss lab and other leaders in the field, a variety of tools have been built on ProteoWizard’s foundations. One such tool is Skyline, an invaluable application for quantitative mass spectrometry.
How has your work making proteomics data more accessible and reproducible influenced the development of the NautilusTM Platform?
Accessibility and reproducibility have been at the forefront of our work since Nautilus’ founding. Knowing the importance of data sharing in the discovery process, we have designed our platform to produce data in the simplest format possible – counts of single protein molecules. We are also building a cloud-based analysis suite that will make it easy for researchers to generate insights from their raw data and produce data visualizations following industry standards. Our goal is to make our platform accessible not only in its sample prep and ease-of-use, but also in its data output and analysis. We hope our efforts will fundamentally accelerate proteomics research and enhance discoveries in basic research, healthcare, and beyond.
MORE ARTICLES
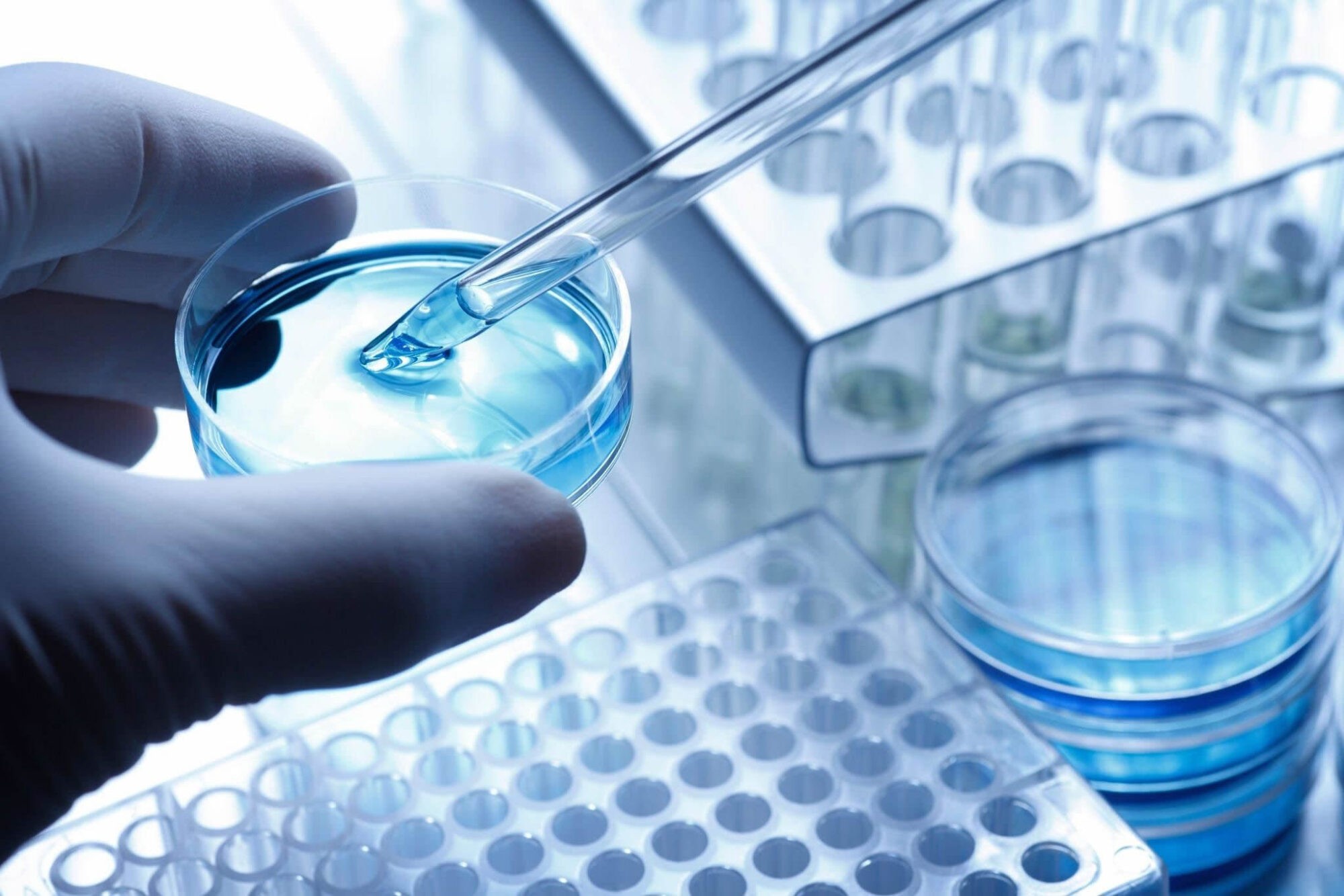