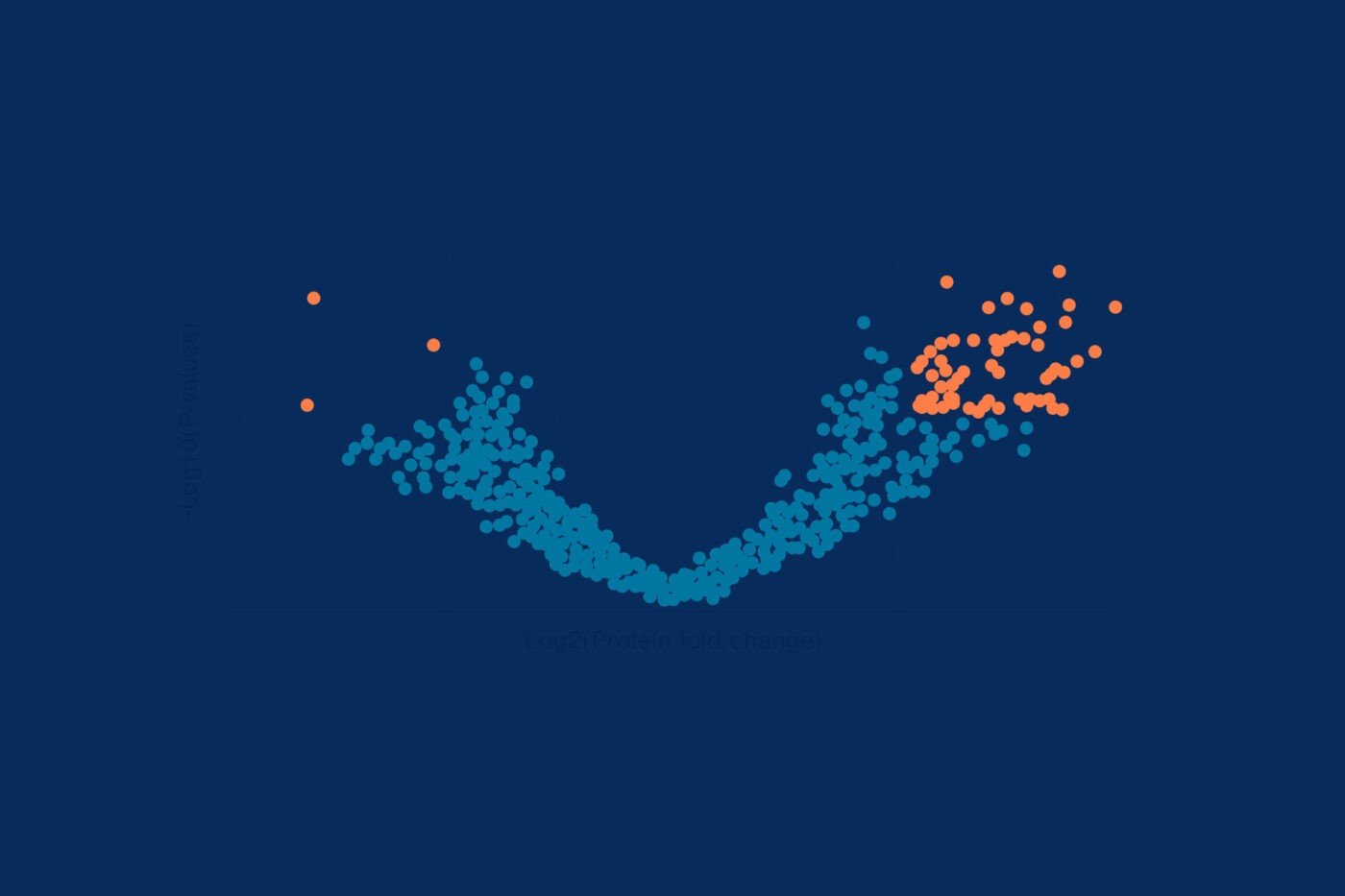
AI in proteomics – Unleashing the potential of artificial intelligence in biomedicine
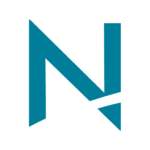
Nautilus Biotechnology
September 24, 2024
It’s no secret that artificial intelligence (AI) is everywhere today, from search engines to writing assistants. There are few domains where experts aren’t predicting the transformative power of AI. Proteomics, and biomedicine in general, is no exception.
There is vast potential for AI to revolutionize how we discover, understand, and even create proteins. The applications of AI in proteomics are likely to begin more modestly, however, and there are real challenges to achieving some of the incredible potential goals of AI-powered proteomics.
In a recent episode of the Translating Proteomics podcast, Nautilus Co-Founder Parag Mallick and Senior Director of Scientific Affairs and Alliance Management Andreas Huhmer offer a grounded perspective on what AI may realistically achieve in proteomics in the next few years, and discuss some of the issues currently holding back future growth. In short, expect AI assistants offering helpful suggestions, not AI super scientists creating brand new proteins from scratch.
That said, the integration of AI and proteomics is not a futuristic concept; it’s happening now, with significant implications for how we understand and manipulate biological systems. Read on for more insights into what that revolution may bring.
AlphaFold: A harbinger of AI’s promise for biology
When AlphaFold, an AI developed by Google’s DeepMind, was unveiled, it was clear that AI had arrived in the world of biology. The system predicts a protein’s three-dimensional structure from its constituent amino acids, a task researchers had long struggled with.
AlphaFold was “the first really compelling use case of AI in biology,” Andreas says.
Insights gained from AlphaFold are already advancing drug discovery, as improved understanding of protein structures is helping identify new therapeutic targets.
However, AlphaFold’s success didn’t happen overnight — it was built on decades of research into protein structure, the development of sophisticated algorithms, and the accumulation of large, well-curated datasets that were essential for training the AI model.
AlphaFold illustrates the potential of AI in biology, while at the same time highlighting the necessity of robust, well-curated datasets. That’s especially true in proteomics.
The challenges of applying AI to proteomics
The protein structure datasets AlphaFold was trained on are relatively well-studied and standardized. In other aspects of proteomics, and biology in general, that’s not always the case. That’s an important point when it comes to creating new AI models for proteomics.
“We can’t fall back on the resources we had for AlphaFold, because we simply don’t have the collection of well-curated datasets in the context of a biological mechanism, for example,” Andreas says.
While databases like PRIDE and ProteomeXchange provide valuable repositories of proteomics data, they’re currently lacking consistency and comprehensiveness, Andreas says. Databases like these contain thousands of datasets, but the data are often collected using different methods, under different conditions, and with varying levels of annotation. On a more basic level, Parag notes, even things like acronyms can present tripping hazards for AI, as different concepts can have the same abbreviations.
Another significant challenge in applying AI to study the proteome is the dynamic nature of proteins and their interactions within biological systems. Proteins do not exist in isolation; they interact with each other and with other molecules in complex networks that are constantly changing in response to various stimuli. To fully leverage AI in this context, we need datasets that capture these temporal and spatial dynamics.
Most existing datasets provide only static snapshots of protein abundance or interactions at a single time point, which limits AI’s ability to model the dynamic processes critical to understanding protein function and disease mechanisms.
Watch Parag and Andreas discuss the challenges of applying AI to biology:
Why now? The perfect storm of data, compute, and algorithms
Given the challenges ahead, why is now the right time to apply AI to biomedicine? Parag outlines three major, intersecting trends that show AI can be a legitimate force in the world of biology:
- The first is simply raw power. “The acceleration we’ve had in GPU compute over the last 10 years has been astonishing,” Parag says. That increase in computational ability has made the number-crunching behind AI models possible on realistic timeframes.
- Second is a vastly expanded capacity for data storage, which has made compiling the kind of massive datasets necessary to train AI possible.
- Finally, the algorithms themselves have improved, and they’ll only get better from here. “There have been some tremendous algorithmic advances in how we represent foundation models, large language models, and more,” Parag says.
Together, these trends are creating AI that is becoming more nimble, intelligent and useful.
Watch Parag and Andreas discuss why now is the time to apply AI to biomedicine:
AI as a tool, not a replacement
Both Parag and Andreas foresee the initial wave of AI in proteomics coming in the form of helpful, efficient assistants, rather than replacements. Parag gives the simple example of a plug-in that can find proteins in UniProt for a researcher as they write a paper.
With such a tool, “our papers are no longer just these static one-dimensional things, but they’re linked to a broader collection of knowledge,” he says.
Other applications will come in areas where patterns in data are subtle, or complex, which might include identifying changes in the abundances of many proteins during disease.
“Machine learning is a tool that ultimately can hack through a lot more data than we can,” Andreas says. They’ll be most useful when “we have to digest huge amounts of data, incredibly complex patterns, and patterns that are probably not consistent enough for us as humans to recognize.”
Another overlooked area where AI could begin assisting researchers, likely with very little in the way of technological advancements, is tracking, organizing, and adding to existing workflows. It’s a concept Parag outlines in his 2024 Gilbert S. Omenn Computational Proteomics Award lecture, where he notes that AI agents could greatly accelerate the iterative cycles of scientific research. The right AI could “continuously accelerate that hypothesize, test, evaluate cycle, and we can quickly run the cycle 100s or even 1000s of times.”
This and other proteomics applications may not yet exist, but they could soon.
Watch Parag and Andreas discuss how AI will impact the study of proteins:
The risks of AI in biomedicine
As with other applications of AI, the use of computer algorithms in biology brings with it risks as well. Most glaring is the danger of bad actors using powerful AI tools to design proteins that could cause harm, in the form of bioweapons. More subtly, AI could be used to interfere with the scientific publishing process by falsifying data or publications. “You could imagine publishing a series of papers saying, ‘oh, hey, this is the coolest, best new thing’ and distracting the scientific community for years,” Parag says.
An overreliance on AI might also sometimes lead to worse outcomes, or cause human skills to atrophy. Parag notes recent research on radiologists using AI to analyze chest X-rays, which found the algorithms didn’t help every radiologist equally, and in fact hurt the performance of some of them.
Watch Parag and Andreas discuss the risks of AI in biomedicine:
Conclusion: Charting the path forward
For the AI revolution to take root in the world of bioscience, several things need to happen, Andreas and Parag say. The first is gathering more data: “We think that we have a lot of data in life sciences, but if we compare it to some of the training sets that large language models use, they were trained over the entirety of Wikipedia or the entirety of the internet,” Parag says.
Ongoing work in so-called “small language models,” could be one solution, though those models are still experimental.
The NautilusTM Proteome Analysis Platform may be an invaluable tool for overcoming AI’s challenges in biomedicine. It is designed to collect comprehensive proteomic data with unprecedented resolution. Alongside other next-generation proteomics tools, Nautilus hopes its platform will contribute to larger, better proteomics databases that can feed future AI models. With that data in hand, AI empowered advances in medicine, protein engineering, and more could come quickly.
MORE ARTICLES
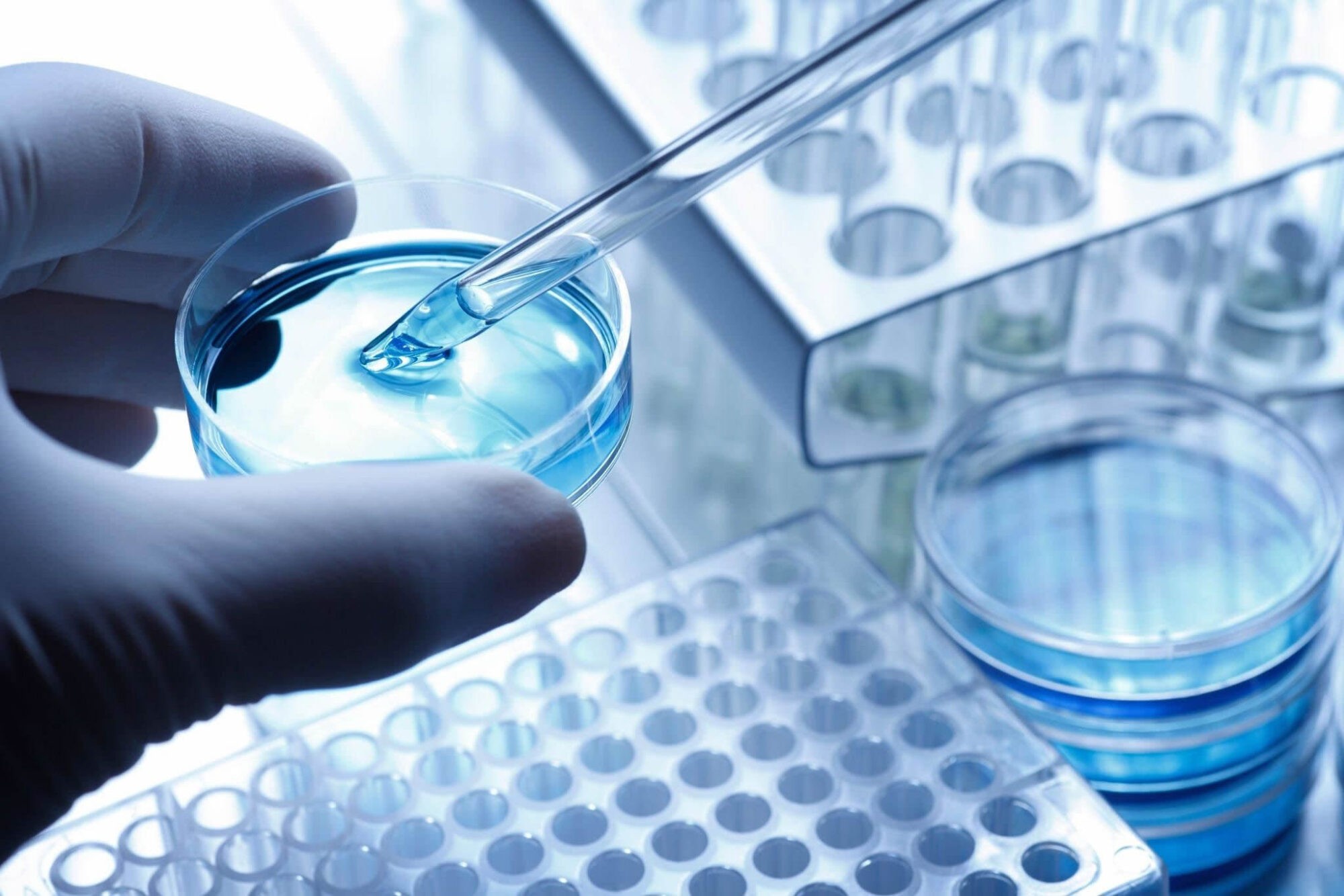