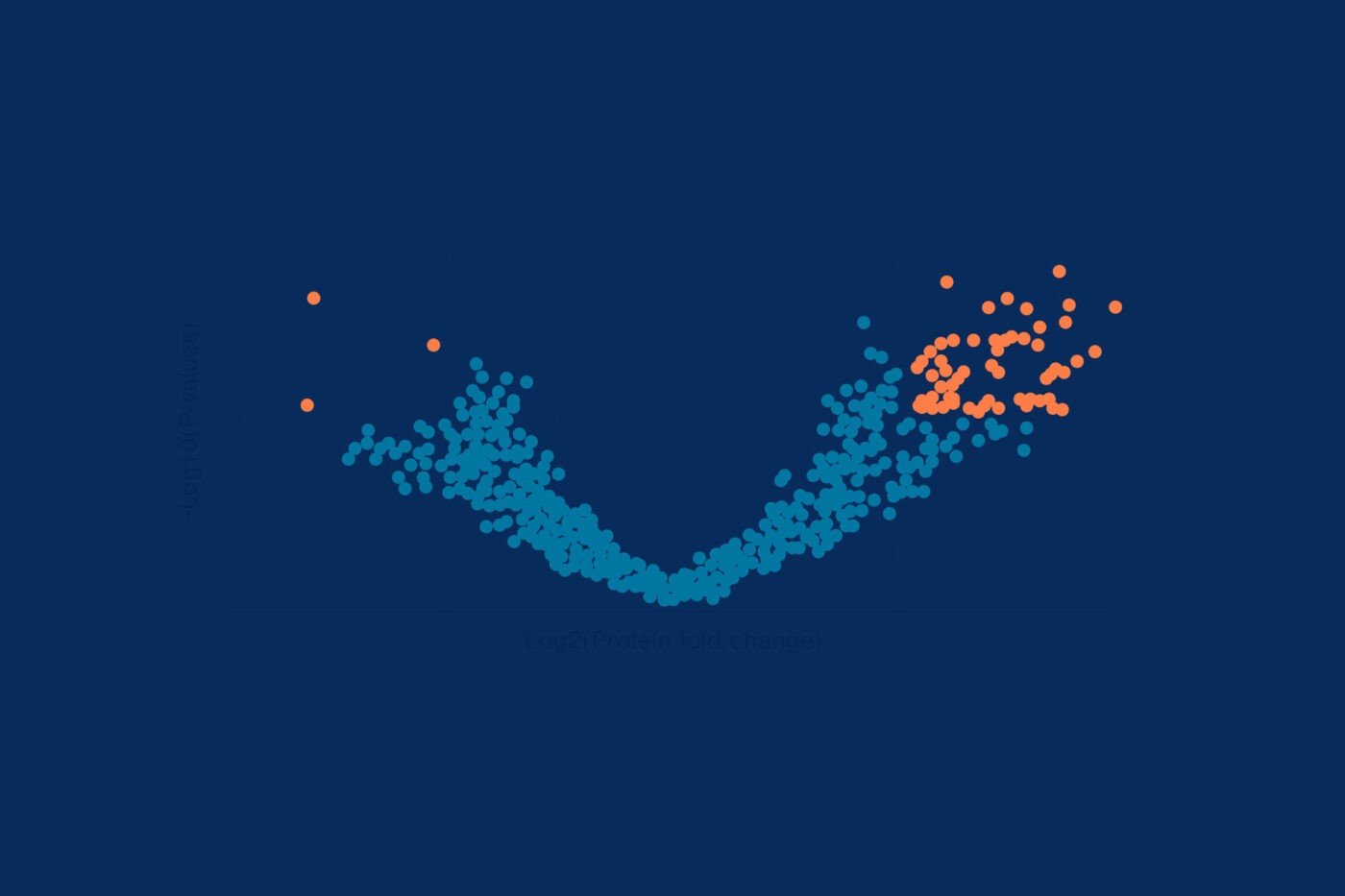
5 ways machine learning impacts modern life (and may even revolutionize biotechnology)
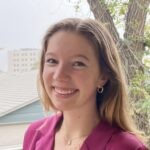
Gracie Murphy
March 30, 2023
Much like the cloud and cryptocurrency, machine learning emerged in modern life without a clear explanation of what it is or why it is necessary. As the name suggests, machine learning refers to the ability of a computer to utilize data and algorithms to mimic human intelligence. Rather than complex code programming a response to every possible scenario, computers learn through experience. By chugging through data – pictures, sounds, even proteins – the machine learns to find patterns, classify things in useful ways, or offer suggestions. While it may seem futuristic, machine learning is a pervasive aspect of modern life that we interact with daily. Smart bots powered by machine learning help us decide what road to take and who to be friends with online. This post dives into a few of the many ways in which machine learning impacts aspects of everyday life and may help ignite a biotech driven future.
Navigation systems – Traffic assessments and ETAs
Have you ever wondered how Google Maps knows the fastest route to get home from work? Or why the Waze app guides you through a maze of backroads instead of the main highway? Whenever you input a destination into a navigation app, an immense amount of data is simultaneously processed to evaluate the fastest route and provide an estimated arrival time. Similar to how seasoned commuters learn to evade traffic bottlenecks, computers learn from past experiences and traffic patterns to guide travelers every day. Not to say that computers will always prevent you from showing up late, but I am guessing you have trusted machine learning to navigate at least once or twice this week.
Speech recognition – “Hey Siri”
Speech recognition software is a prime example of machine learning. Siri functions by listening to our requests, processing that information, and presenting an applicable response. Simple right? Not exactly. Being able to recognize speech, both words and tone, is much more difficult than it may appear. To decipher the nuances behind human language, Siri learns to recognize characteristic frequencies and sound waves to piece together spoken words and phrases. As with understanding any new language, Siri learns through ample exposure to speech and practice, becoming the comforting robotic presence we recognize today.
Social Media – People you may know
Remember that kid from your high school biology class? Facebook does! Instagram also found your middle school crush and your distant cousin from Nebraska in case you are interested in reconnecting. Social media has learned to make recommendations regarding friendships and even acquaintances to an almost spooky level. Social media does this by sifting through your own online friends, and their online friends, and their online friends to compile an extensive list of people you may know based on shared connections, employers, alma maters, or even hobbies. As your online network grows and your network’s network grows, social media sites like Instagram and Facebook uncover more hidden connections – some of which we may be more grateful for than others.
AlphaFold – Protein structure
Although proteins are an essential component of living things, only a fraction of protein structures have been uncovered, limiting existing knowledge regarding protein function. AlphaFold surpasses current laborious processes of protein structure identification, using a computational method to accurately infer protein structures. This system works by combining existing knowledge regarding known protein structures, thermodynamics of protein folding, evolutionary history, and geometric constraints of protein structures. This effective use of machine learning hopes to catalyze structural bioinformatics and become a staple modern biology tool.
Machine learning as a driving force behind the proteomics revolution
If you have spent some time poking around the Nautilus Blog, you may already know that the Nautilus Proteome Analysis Platform utilizes machine learning to identify and quantify individual proteins in a sample. The Nautilus platform works by interrogating proteins with hundreds of fluorescent multi-affinity probes, with each probe uncovering a little more information about each protein in the sample. The machine learning analysis software is designed to decipher all this binding information into actual protein identifications. Founder Parag Mallick has described this process as a game of protein Guess Who. The software is designed to enable the identification of billions of proteins at once, an unprecedented expansion in the scope of proteome coverage.
The actual computer science underlying Nautilus’ technology, Google Maps, Siri, Facebook, and AlphaFold is incredibly complex, but they share a similar principle – synthesizing massive amounts of data combined with past experiences to computationally classify a variety of things in an educated way. We may still be years away from computers learning how to think and feel just like us, but for now we can appreciate the ways in which machine learning makes our commutes, our research, and our lives a little (or a lot) easier.
MORE ARTICLES
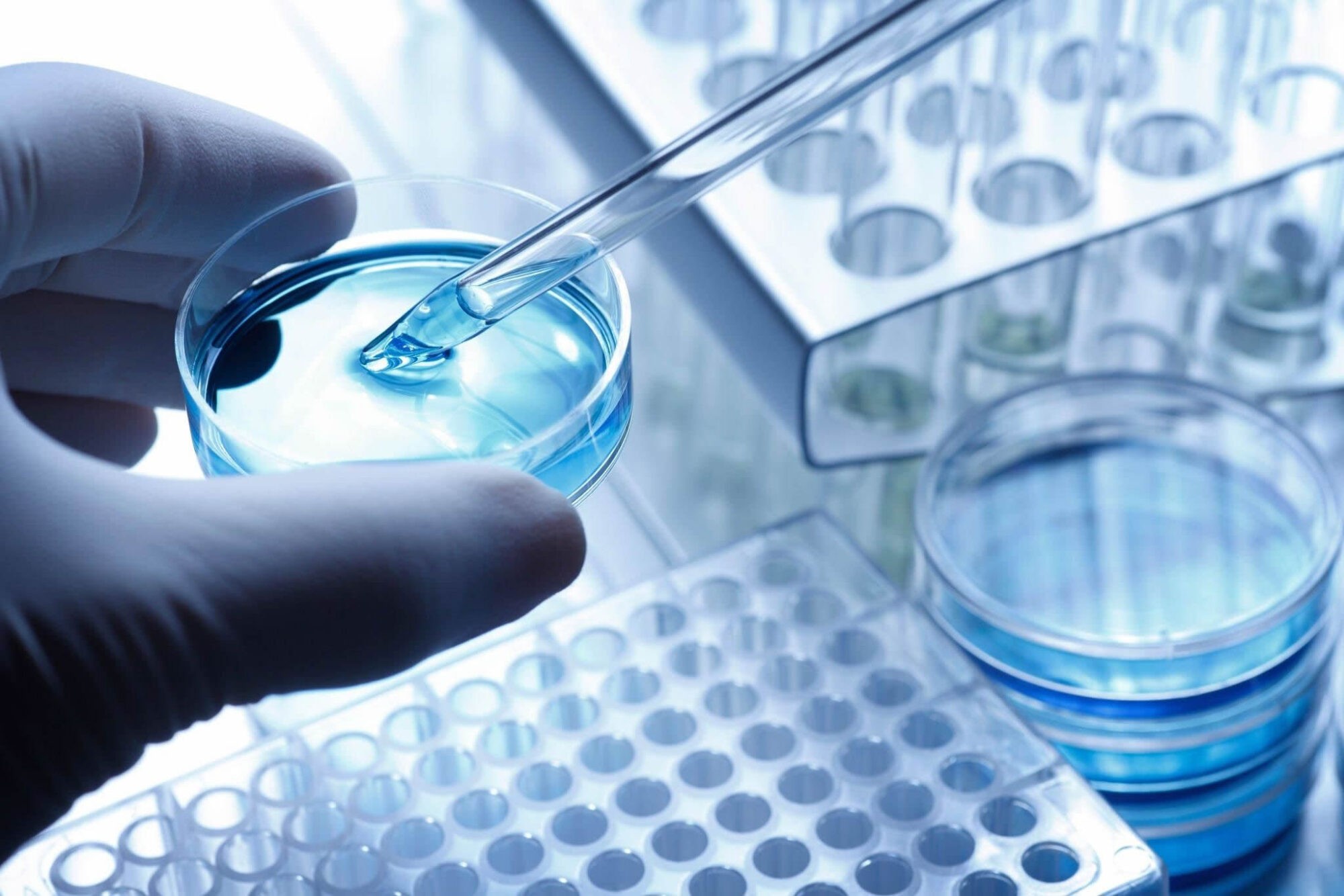